Artificial intelligence is here. What do I need to know?
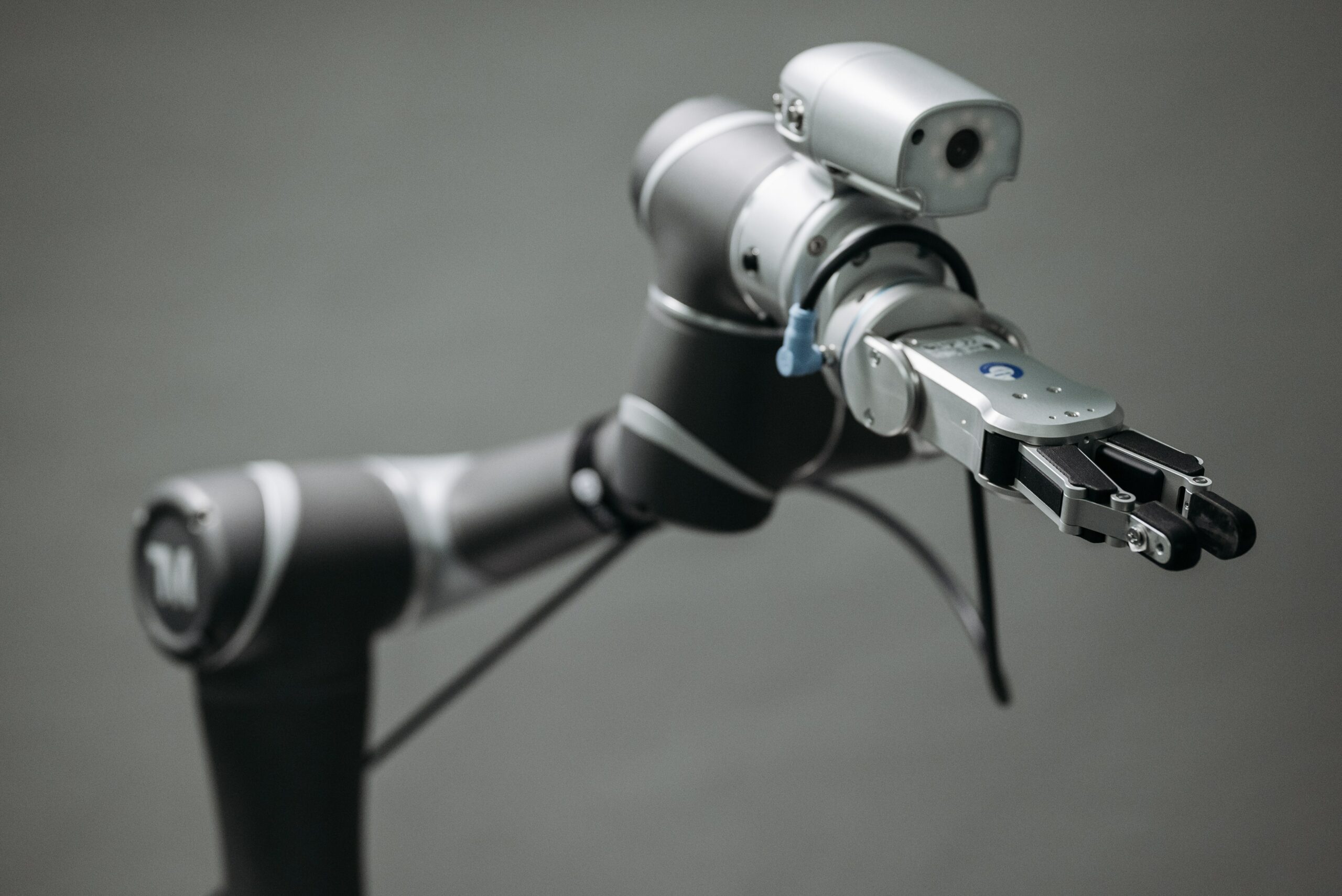
As AI develops, we reshape our expectations based on what’s currently possible, and what we’d like to solve next
There’s been a lot of excitement about ‘ChatGPT’ or ‘the new Bing’. And if you watch any big tech company talk about a new product you’ll inevitably hear the buzzwords ‘machine learning’ and how it can unlock new possibilities.
Whether it’s in our personal lives, or in large services delivered by the public sector, expectations are growing around the possibilities of AI.
What is AI?
Defining AI is difficult. At different points in time, we think something is AI, but then we find something more complex, and that old technology feels a lot less intelligent. Deep Blue, a chess bot, handily defeated chess grandmaster Garry Kasparov in 1997. While stunning at the time, few of us would now think of ‘chess bots’ as the vanguard of technology.
As AI develops, we reshape our expectations based on what’s currently possible, and what we’d like to solve next.
In the most general sense, AI is intelligence delivered by technology, as opposed to humans. The way it achieves this will vary on the method, but generally relies on data and a lot of training. The difference between a regular piece of software and something that uses ‘artificial intelligence’, is that the latter will learn and improve without being explicitly told to do so. That sometimes means improving over a long period of time, or it could mean a human correcting the AI and narrowing their request down with more information.
Ultimately, the AI has to do some background work – gather more data, keep trying, adjust and improve. To achieve this, they need to have a way to learn.
Supervised learning
Some programs are trained via supervised learning, where humans tag data before it’s ingested by an algorithm (a set of instructions). Here’s an example.
Imagine you want a robot to make you a coffee. If you let it into your kitchen without any instructions, there’s going to be havoc. So you need to tell it where the coffee is, where the water is, where the milk is, and so on. This means that instead of checking every single item in your kitchen, and wasting all your tea bags before eventually finding the coffee, it can begin its work with the tagged item from the start.
Through tagging data, we make it possible for a machine to quickly read through data and take some kind of action on it. The robot could still surprise me by picking a different kind of coffee, but it’s already got a foundation of knowledge from which it can make decisions.
A great example of this is the spam filter in your email box. Thanks to massive datasets, big companies like Google and Microsoft can take all the learning from previous spam emails and automatically keep them out of your inbox (well, most of the time). If you report a new email as spam – congrats, you’ve just tagged some more data for the AI!
The problem with supervised learning is that it takes quite a bit of upfront effort. Depending on your budget, the time available, and the sheer processing power available (human or machine), this might not be a workable solution.
Unsupervised learning
Another route to try is unsupervised learning. Unlike supervised learning, you put the onus on the machine to find a pattern. The risk here is that it might not find what you were looking for, but where you have data that is messy, or a problem area that’s poorly understood by humans already, this can reveal patterns that lead to breakthroughs.
Making a coffee is a pattern that’s pretty well understood by humans and easy to program into a set of instructions. Does it make my life easier for a robot to do it? Sure, but I can still make coffee if I have to. The real value lies in the application of AI to difficult problems, such as complex cases in medicine.
Radiologists are great at finding most tumours early enough for a health service to take action – but what about the cases we miss? This is where an AI based on unsupervised learning can really shine. We don’t need an AI to find the 90%+ of cases humans already catch, we’re just rolling a dice to see if it can catch the remaining 10%. If we combine the abilities of an AI that can find irregular elements that wouldn’t be perceptible to the human eye, the final check by a radiologist leads us to a rate that’s much closer to 100%.
Ultimately, artificial intelligence’s usefulness depends on the problem we’re trying to solve – sometimes it’s worth letting the AI do the exploration we can’t. If you imagine a dark night sky, an AI trained on supervised data could easily pick out major star formations or the planets of the solar system. An AI trained on unsupervised data, however, might notice objects not usually there – a distant meteor passing through or maybe a one-off visitor from afar!
The relative magic of AI is still linked to what we want to do with it, and doesn’t remove the ethical challenges we already face as humans.
How can it go wrong?
When testing ChatGPT in recent weeks, many users were delighted at its helpful responses, that is, until it got into a disagreement. Frustrated as it felt it knew better, ChatGPT got more and more abrupt before cutting off the conversation. The problem is that storming out of an argument is a very human thing to do!
The language these models use mimic the data they are trained on, and it turns out basing that on the whole internet can sometimes turn sour.
For bigger, complex problems, this poses a real challenge. You become more able than ever to churn through massive amounts of data and get a more reflective answer than any one human can, but you’re still stuck with the limits of a biased dataset.
Imagine you want to use AI to help ease a backlog of medical cases. The AI could be a real gift, quickly flicking through historical data and finding patterns that may relate to a patient’s current case. Have you been here a few times this year? Well maybe you have an underlying condition, let’s do a blood test. Got a case where a patient has ended up in A&E after missing a GP appointment? Let’s link them up and see if the symptoms seem related to issues they’ve reported before.
The problem lies in the data we already have. If the medical system already has shortcomings that let down particular groups, such as biases against women or people of colour reporting symptoms, an AI is likely to replicate those biases.
Want an appointment? We’re too busy and it doesn’t sound serious enough. Who said it’s not serious enough? Well, all the previous data we’ve got.
When applying technology to big, complex systems and problems, we have to really think about what we’re trying to achieve. Do you want the most efficient allocation of appointment times? The prioritisation of the most urgent cases? The more questions you ask for implementing artificial intelligence, the more decisions come back to humans.
What AI can’t do
Across the public sector, the challenge in doing clever things with technologies like artificial intelligence lies with the outcomes we’re looking for. You can throw AI at all sorts of problems, but to make great things for people, you also have to think deeply about what’s not working already and actively design around it.
AI will make it easier for us to automate processes and to free up human time for complex problems. AI will make it easier to discover patterns that we can’t work out by ourselves. Those are exciting possibilities that are already being taken advantage of in the public sector, and good design will have to take into account the new ways we can design and deliver for users.
Despite these developments, AI alone can’t design for people. It can do its best to imitate good design, or at the very least what’s been done before, but it’s still up to us to make complex decisions and create brilliant things.
If you’d like to read more about how algorithms and AI work, as well as some of the broader ethical challenges of using technology like this, I’d recommend Hello World: How to be Human in the Age of the Machine by Hannah Fry.
Featured image by Pavel Danilyuk